.sticker-float[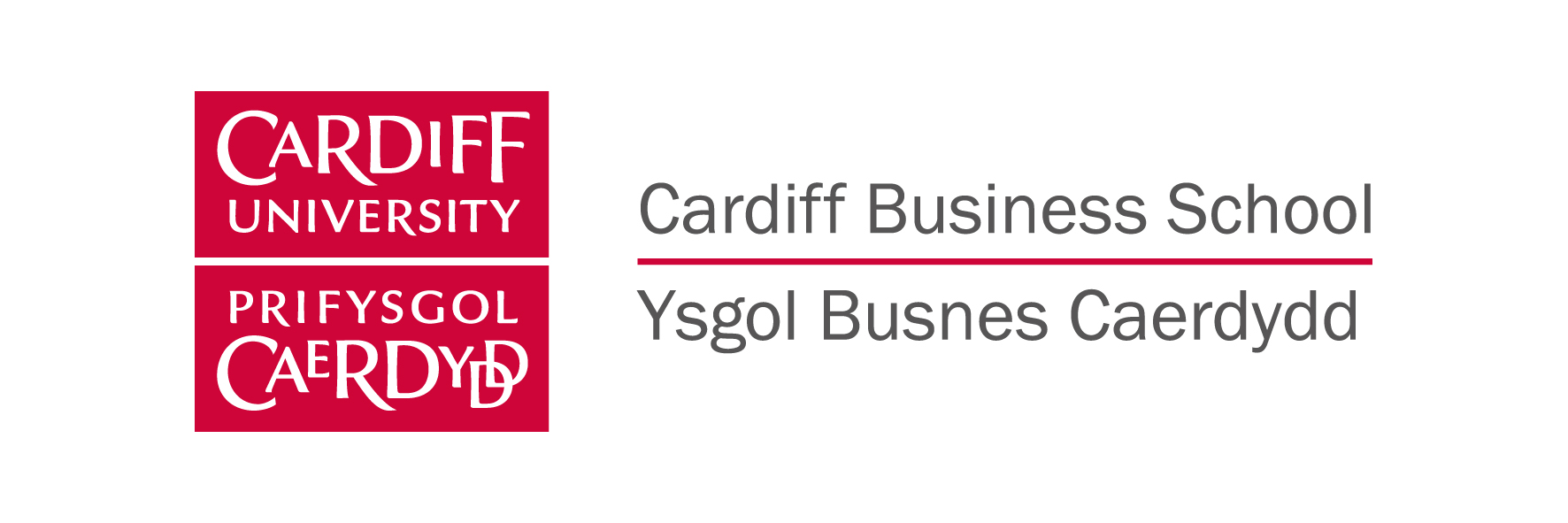] <br><br> .center[.title[Hierarchical forecast reconciliation for vaccine demand]] .bottom[ Collaboration with JSI <br> Bahman Rostami-Tabar, Reader in Data & Management Science
[rostami-tabarb@cardiff.ac.uk](rostami-tabarb@cardiff.ac.uk)
[www.bahmanrt.com](https://www.bahmanrt.com/) ] --- background-image: url("resources/hierarchy-left.jpeg") background-size: contain background-position: left class: middle .pull-right2[ ## Outline - Immunization supply chains - Forecasting problem - Forecasting experiment setup - Forecast accuracy evaluation - Conclusions & next steps ] --- background-image: url("resources/hierarchy-left.jpeg") background-size: contain background-position: left class: middle .pull-right2[ ## Outline - .remember[Immunization supply chains] - .gray[Forecasting problem] - .gray[Forecasting experiment setup] - .gray[Forecast accuracy evaluation] - .gray[Conclusions & next steps] ] --- class: center, middle .pull-left[ <img src="img/f4sg2022_vaccine/children_immunization.png" height="350px"> ] .left[ .pull-right[ - Approximately .remember[1 in 5] African children .remember[do not receive] all basic vaccines. - More than .remember[30 million children] under five still .remember[suffer from vaccine-preventable diseases (VPDs)] every year in Africa - Over .remember[half a million children die] from VPDs annually - Logistics and Operation Managements contributes to this pressing issue. ]] .footnote[source: [**WHO**](https://www.afro.who.int/health-topics/immunization]) --- ## What do we want to achieve? .pull-left[ ### High coverage <img src="img/f4sg2022_vaccine/coverage.jpg" height="500px"> ] -- .pull-right[ ✅ Reduce stock outs ✅ Reduce missed opportunities ✅ Lower waste ✅ Lower inventory costs ✅ and better coordination ] .footnote[source:[Allan etal.(2021)](https://www.medrxiv.org/content/10.1101/2021.02.14.21251721v1.full) ] --- class:inverse, center, middle .title[ Forecasting accurately the needs for vaccines is one of the key elements in achieving these goals] --- ## The immunization supply chain <img src="img/f4sg2022_vaccine/vaccine_supply_chain.png" height="540px"> .footnote[Source: Effective Vaccine Management Assessment (EVM), 2021] --- ## Vaccines .three-column[ ### Vial <img src="img/f4sg2022_vaccine/vial.jpg" height="600px"> ] -- .three-column[ ### Dose <img src="img/f4sg2022_vaccine/dose.jpg" height="600px"> ] -- .three-column[ ### Administrated <iframe src="https://giphy.com/embed/xT5LMGfQrJPpmXKUEM" height="600px" allowFullScreen></iframe><p><a href="https://giphy.com/gifs/season-16-the-simpsons-16x11-xT5LMGfQrJPpmXKUEM">via GIPHY</a></p> ] --- .center[ .pull-left[ ### Open wastage <img src="img/f4sg2022_vaccine/open_waste.jpeg" height="600px" > ] .pull-right[ ### Close wastage <img src="img/f4sg2022_vaccine/vaccine_wastage.jpeg" height="600px"> ] ] --- class: inverse, center, middle .title[Doses used (consumption/needs) <br> = <br>doses administrated + wastage] --- background-image: url("resources/hierarchy-left.jpeg") background-size: contain background-position: left class: middle .pull-right2[ ## Outline - .gray[Immunization supply chains] - .remember[Forecasting problem] - .gray[Forecasting experiment setup] - .gray[Forecast accuracy evaluation] - .gray[Conclusions & next steps] ] --- ## Map of Kenya .center[ <img src="img/kenya.png" height="450px"> ] --- ## Hierarchical structure of vaccine data .center[ <img src="img/f4sg2022_vaccine/hierarchy.png" height="380px"> ] --- ## Classical approaches to forecast vaccine needs - Demographic methods (developed by WHO, one-size-fits-all model) - expected target population - coverage: is the expected coverage rate - doses/target: is the number of doses per target, as per the national vaccination schedule - wastage factor .alert-note[.remember[**Forecasts are annual and at the national level**]] --- ## Limitations of current forecasting method ❌ Forecasts are yearly and at the national level(not useful to inform operational / tactical decisions.) ❌ Forecasts are often produced based on unrealistic assumptions. ❌ Forecasts do not acknowledge uncertainty. ❌ Do not capture the information available at multiple hierarchical levels. ❌ Forecasts ignore the hierarchical nature of the problem, not coherent. ❌ Lead to conflicting decisions & lack of coordination. --- ## How to forecast hierarchical time series? .pull-left[ - Base forecast (generated using any forecasting model) - Bottom-Up - Top Down - Middle-Out - .remember[Forecast reconciliation] ] .pull-right[ <img src="img/f4sg2022_vaccine/pyramid.jpg" width="90%" style="display: block; margin: auto;" /> ] --- background-image: url("resources/hierarchy-left.jpeg") background-size: contain background-position: left class: middle .pull-right2[ ## Outline - .gray[Immunization supply chains] - .gray[Forecasting problem] - .remember[Forecasting experiment setup] - .gray[Forecast accuracy evaluation] - .gray[Conclusions & next steps] ] --- ## Data - Vaccine consumption in Kenya from January 2013 until December 2021 - Four type of vaccines - Measles - Bacillus Calmette–Guérin (BCG) - for tuberculosis - DPT, a class of combination vaccines against three infectious diseases in humans: diphtheria-tetanus-pertussis - OPV, Oral poliovirus vaccines, used in the fight to eradicate polio - 306 sub-county, 47 county & 9 regions - Total of 1452 time series --- ## Doses used (raw data): total and regions <img src="img/f4sg2022_vaccine/dose_used.png" height="500px"> --- ## Doses used: National & regions .center[ <img src="img/f4sg2022_vaccine/p1.png" height="600px"> ] --- ## Doses used: trend and seasonality features .center[ <img src="img/f4sg2022_vaccine/admin_hts_feature_cat_plot.png" height="600px"> ] --- ## Forecasting setup - Forecasting method: Exponential Smoothing State Space models - Forecast horizon: 12 months - Point and probabilistic forecasts are generated and evaluated for the entire hierarchy - Forecast evaluation - Time series cross-validation with re-estimation - Used 36 months as test set --- ## Forecasting performance metrics- point forecast .pull-left[ ### Mean Absolute Scaled Error `$$\large \text{MASE} = \text{mean}(|q_{j}|),$$` where `$$q_{j} = \frac{ e_{j}} {\displaystyle\frac{1}{T-m}\sum_{t=m+1}^T |y_{t}-y_{t-m}|},$$` ] .pull-right[ ### Mean Squared Scaled Error `$$\large \text{MSSE} = \text{mean}(q_{j}^2),$$` where, `$$q^2_{j} = \frac{ e^2_{j}} {\displaystyle\frac{1}{T-m}\sum_{t=m+1}^T (y_{t}-y_{t-m})^2},$$` ] --- ## Forecasting performance metrics- probabilistic forecast .pull-left[ `$$\large \text{CRPS} = \text{mean}(p_j),$$` where `$$p_j = \int_{-\infty}^{\infty} \left(G_j(x) - F_j(x)\right)^2dx,$$` ] .pull-right[ <img src="figs/crps.jpg" height="450px"> ] --- background-image: url("resources/hierarchy-left.jpeg") background-size: contain background-position: left class: middle .pull-right2[ ## Outline - .gray[Immunization supply chains] - .gray[Forecasting problem] - .gray[Data of vaccine consumption & forecasting setup] - .remember[Forecast accuracy evaluation] - .gray[Conclusions & next steps] ] --- ## Overall forecast accuracy .small[ <table class=" lightable-classic" style="font-family: Cambria; width: auto !important; margin-left: auto; margin-right: auto;"> <caption>MSSE</caption> <thead> <tr> <th style="text-align:left;"> Method </th> <th style="text-align:right;"> Total </th> <th style="text-align:right;"> Region </th> <th style="text-align:right;"> County </th> <th style="text-align:right;"> Sub County </th> </tr> </thead> <tbody> <tr> <td style="text-align:left;"> Base </td> <td style="text-align:right;"> 0.526 </td> <td style="text-align:right;"> 0.594 </td> <td style="text-align:right;"> 0.656 </td> <td style="text-align:right;"> 0.651 </td> </tr> <tr> <td style="text-align:left;"> Bottom Up </td> <td style="text-align:right;"> 0.509 </td> <td style="text-align:right;"> 0.594 </td> <td style="text-align:right;"> 0.645 </td> <td style="text-align:right;"> 0.649 </td> </tr> <tr> <td style="text-align:left;"> Top Down </td> <td style="text-align:right;"> 0.526 </td> <td style="text-align:right;"> 0.807 </td> <td style="text-align:right;"> 0.739 </td> <td style="text-align:right;"> 0.717 </td> </tr> <tr> <td style="text-align:left;"> Reconciliation </td> <td style="text-align:right;"> 0.428 </td> <td style="text-align:right;"> 0.510 </td> <td style="text-align:right;"> 0.600 </td> <td style="text-align:right;"> 0.609 </td> </tr> </tbody> </table> <table class=" lightable-classic" style="font-family: Cambria; width: auto !important; margin-left: auto; margin-right: auto;"> <caption>CRPS</caption> <thead> <tr> <th style="text-align:left;"> Method </th> <th style="text-align:right;"> Total </th> <th style="text-align:right;"> Region </th> <th style="text-align:right;"> County </th> <th style="text-align:right;"> Sub County </th> </tr> </thead> <tbody> <tr> <td style="text-align:left;"> Base </td> <td style="text-align:right;"> 349.345 </td> <td style="text-align:right;"> 100.880 </td> <td style="text-align:right;"> 55.236 </td> <td style="text-align:right;"> 30.256 </td> </tr> <tr> <td style="text-align:left;"> Bottom Up </td> <td style="text-align:right;"> 320.343 </td> <td style="text-align:right;"> 89.345 </td> <td style="text-align:right;"> 45.223 </td> <td style="text-align:right;"> 29.341 </td> </tr> <tr> <td style="text-align:left;"> Top Down </td> <td style="text-align:right;"> 374.431 </td> <td style="text-align:right;"> 105.123 </td> <td style="text-align:right;"> 66.345 </td> <td style="text-align:right;"> 51.340 </td> </tr> <tr> <td style="text-align:left;"> Reconciliation </td> <td style="text-align:right;"> 256.345 </td> <td style="text-align:right;"> 74.345 </td> <td style="text-align:right;"> 36.234 </td> <td style="text-align:right;"> 23.654 </td> </tr> </tbody> </table> ] .footnote[Forecast accuracy improvement against the current approach in immunization programs is calculated but not included in the presentation] --- background-image: url("resources/hierarchy-left.jpeg") background-size: contain background-position: left class: middle .pull-right2[ ## Outline - .gray[Immunization supply chains] - .gray[Forecasting problem] - .gray[Forecasting experiment setup] - .gray[Forecast accuracy evaluation] - .remember[Conclusions & next steps] ] --- ## Conclusions ### Benefit of hierarchical forecasting ✅ Plans at any level are based on .remember[coherent forecasts] and therefore can be aligned. ✅ Hierarchical forecasting framework can be used as a tool to .remember[improve coordination] between teams across the supply chain at the national, sub-national, regional and local levels. ✅ Result can be .remember[more accurate than the independent (base) forecasts]. ✅ Hierarchical forecasting framework an be used to create coherent forecast, regardless of how base forecasts are created, even with judgmental forecasts. --- ## Next steps - Collect data on potential useful predictors such as child population, conflicts, strike, weather conditions, etc - Build new forecasting models incorporating strong driving factors of vaccine demand - Evaluate the implication of forecast accuracy on utilities such as cost, service level, coordination, etc by linking to replenishment policies. --- ## Acknowledgement - John and Snow Inc. (JSI) team - The representative of the National Vaccine Immunization Program in Kenya - [Developers of Tidyverts](https://tidyverts.org/) and [Tidyverse](https://www.tidyverse.org/) packages --- ## References - Effective Vaccine Management (EVM 2.0) Assessment Report – 2021, WHO - Vaccine Logistics, WHO training manual - - [Probabilistic Forecast Reconciliation For Emergency Services Demand]([https://robjhyndman.com/seminars/fem.html], (Bahman Rostami-Tabar, Rob J Hyndman] - [Forecasting: Principles and Practice]([https://otexts.com/fpp3/hierarchical.html], (3rd ed, Rob J Hyndman and George Athanasopoulos] --- .pull-left[ ## About me Bahman Rostami-Tabar <br> Associate Professor in Data and Management Science Cardiff University, UK <br><br> Slides @ [www.bahmanrt.com](www.bahmanrt.com)
[@Bahman_R_T](https://twitter.com/Bahman_R_T)
Connect: [Bahman ROSTAMI-TABAR](https://www.linkedin.com/in/bahman-rostami-tabar-1046171a/) ] .pull-right[ ## Outline of my talk - Immunization supply chain - Forecasting problem - Forecasting experiment setup - Forecast accuracy evaluation - Conclusions & next steps ] --- ## Linear reconciliation methods, Bottom-up and others Forecast reconciliation approaches combine and reconcile all the base forecasts in order to produce coherent forecasts. -- Linear reconciliation methods (Wickramasuriya, Athanasopoulos, and Hyndman 2019) can be written as `$$\large \tilde{{y}}_h = {S}({S}'{W}^{-1}{S})^{-1}{W}^{-1}\hat{{y}}_h ={S}{G}\hat{{y}}_h = {M}\hat{{y}}_h,$$` where `\({W}\)` is an `\(n \times n\)` positive definite matrix, and `\(\hat{{y}}_h\)` contains the `\(h\)`-step forecasts of `\({y}_{T+h}\)` given data to time `\(T\)`. -- .small[ - Different choices for `\({W}\)` lead to different solutions such as Ordinary Least Squares (OLS), Weighted Least Squares (WLS) and Minimum Trace (MinT). - We use the implementation of these methods in the `hts` package in R in the experiment. ] --- ## Producing probabilistic forecasts - We use bootstrapping to generate probabilistic forecasts: - Suppose that `\((\hat{{y}}_h^{[1]},\dots,\hat{{y}}_h^{[B]})\)` are a set of `\(B\)` simulated sample paths, generated independently from the models used to produce the base forecasts. - Then `\(({S}({S}'{W}^{-1}{S})^{-1}{W}^{-1}\hat{{y}}_h^{[1]},\dots,{S}({S}'{W}^{-1}{S})^{-1}{W}^{-1}\hat{{y}}_h^{[B]})\)` provides a set of reconciled sample paths, from which percentiles can be calculated. --- class: center ## Probabilistic forecast .center[ <img src="figure/wast_hiararchy/daily_probabilistic_forecast.png" height="550px"> ] --- ## Reconciled forecasts - This approach involves first generating independent base forecast for each series in the hierarchy (i.e. Base) -- - As these base forecasts are independently generated they will not be “aggregate consistent” (i.e., they will not add up according to the hierarchical structure). They are .remember[not coherent]. -- - The reconciliation approaches combine the independent base forecasts and generates a set of revised forecasts that are as close as possible to the univariate forecasts but also aggregate consistently with the hierarchical structure. -- - Unlike any other existing method, this approach uses all the information available within a hierarchy.